After spending the day at Big Data LDN a few weeks back, I put together a blog article about my three big takeaways for the show.
One of the biggest focal points for me was the importance of making sure your data is fit-for-purpose when considering utilising AI within your business… so I decided to elaborate on that point.
In short, the quality of your data is THE most important consideration for AI.
Whether you’re working with large language models (LLMs) or generative AI, clean and uniform data is the foundation upon which successful AI applications are built.
Why is it that important?
Accuracy and Reliability
Clean data ensures that the AI models you develop are accurate and reliable. When data is free from errors, inconsistencies, and duplicates, AI algorithms can learn more effectively. This leads to more precise predictions, better decision-making, and overall improved performance of AI systems.
Efficiency in Training
Uniform data simplifies the training process for AI models. When data is standardised, it reduces the complexity of processing and allows for more efficient training. This not only saves time, but also reduces computational resources, making the development process more cost-effective when considering cost per consumption models like Azure or AWS.
Enhanced Model Performance
AI models, especially LLMs and generative AI, thrive on large volumes of high-quality data. Clean and uniform data helps in minimising noise and irrelevant information, allowing the models to focus on learning meaningful patterns. This results in enhanced model performance and more accurate outputs.
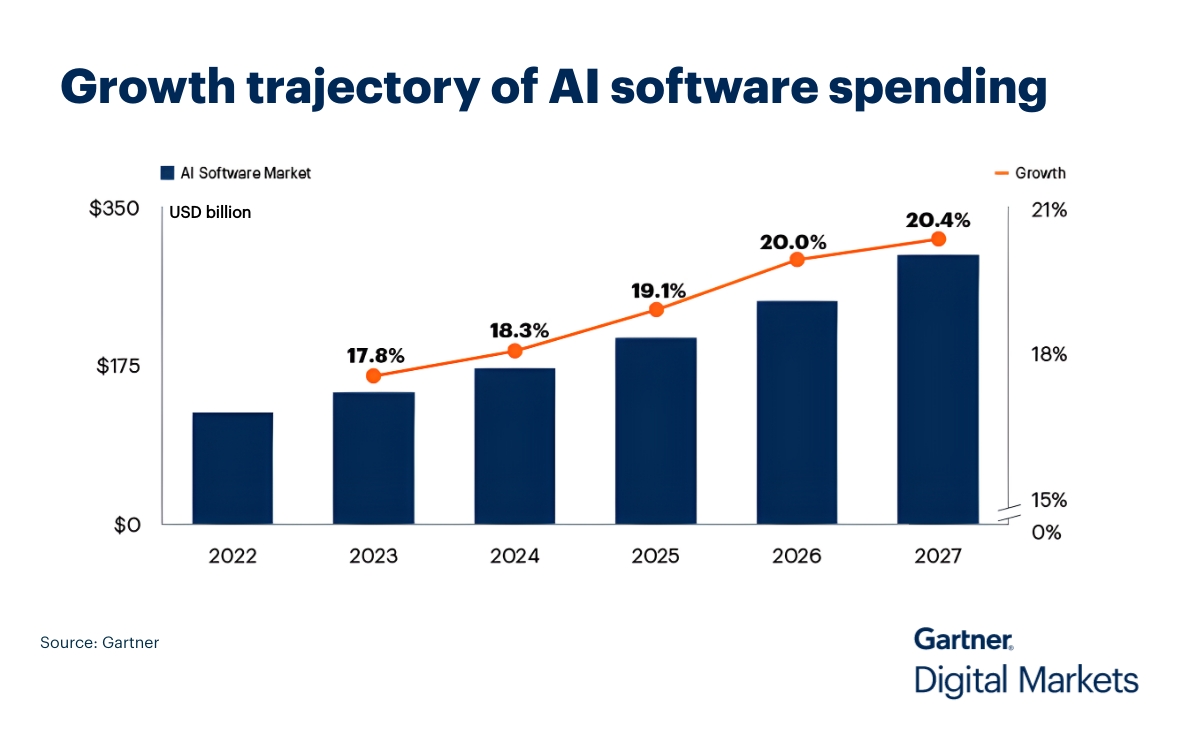
Gartner is predicting nothing other than the contiuned increase of spending on AI projects
Scalability
As AI applications scale, the importance of clean data becomes even more pronounced. Uniform data structures enable seamless integration and scalability of AI systems. This is particularly important for businesses looking to expand their AI capabilities across different departments or regions.
Ethical AI Development
Maintaining clean and uniform data is also a step towards ethical AI development. It helps in reducing biases that can arise from flawed or inconsistent data. By ensuring that the data used to train AI models is representative and unbiased, we can develop AI systems that are fairer and more inclusive.
Decision Making (the big one!)
For me, this is one of the most important aspects of the whole piece. Organisations make huge business critical decisions based on data, and nothing is going to change that any time soon. As much as we’ll come to rely on AI doing the legwork, ultimately it will be someone just like you or I say in a chair making a call based on the output.
So, what happens if your AI model has had bad data to consider?
You guessed it – absolute rubbish.
Sort your data out at the source and you negate this as an issue off the bat.
To close…
The power of AI, particularly LLMs and generative AI, is significantly amplified by the quality of the data they are trained on. Clean and uniform data not only enhances the accuracy and efficiency of AI models but also supports ethical and scalable AI development. As we continue to push the boundaries of what AI can achieve, prioritising data quality will remain a critical factor in unlocking its full potential.
by Steve Clarke – Commercial Director at The Ark
The Ark specilises in data cleansing, single customer view, and identity resolution. If you’d like to speak to us about any of these topics, get in touch today.
.